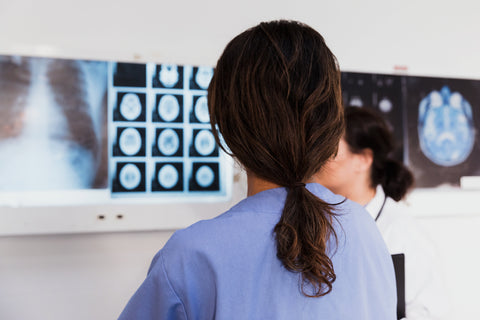
Predicting Myocardial Infarctions and Strokes in Clinical Trials Patients
Predicting Myocardial Infarctions and Strokes in Clinical Trials Patients
The Problem:
Our client was a pharmaceutical company who develops drugs for patients with heart disease and tests those drugs through clinical trials. During the course of a clinical trial, a patient may become admitted to the hospital for any number of reasons. Often the reason for hospitalization is directly related to the drug being tested, such as in the case of a myocardial infarction or a stroke. In fact measurement of the frequency of these events between the test group and the control group can often be a key metric of the drug's efficacy. The challenge is that once a patient is hospitalized, there is a long and complex process for the clinical trial administrators to collect all medical records and have the event adjudicated by multiple cardiologists independently. This process typically takes over four months to determine whether or not a hospitalization is a relevant event or not.
The Data:
The client provided health records for multiple clinical trials, each trial consisting of around 20,000 patients over the course of three years. These records include demographics, lifestyle information, medical history, prescribed medications, and other relevant EMR data. They also included the time and type of event in the case of myocardial infarctions and strokes.
The Solution:
We extracted relevant features and built a Random Survival Forest model, which could predict the risk score for each patient over the course of the trial. The model's overall classification accuracy was over 80%, which exceeded the client's expectations.
The Impact:
Whenever a patient is identified to be hospitalized, the client can now use this model to assess the likelihood that they had a myocardial infarction or stroke, and prioritize patient investigations accordingly. Over the course of the project we also identified a secondary use case, where the client can use this model as a screening tool when selecting patients for new clinical trials.